As online platforms continue to grow, ensuring user safety and preventing the spread of harmful content has become a critical priority. This guide provides platform operators, and content moderators with practical information about CSAM detection tools and how to implement them effectively.
Understanding CSAM
Before exploring CSAM detection tools, it's essential to understand the distinction between known and unknown CSAM, as this determines which detection technologies will be most effective.
Known CSAM
Known CSAM consists of previously identified, verified, and catalogued instances of child sexual abuse material. These materials are converted into cryptographic hashes and stored in specialized databases. This approach enables detection while ensuring the actual content is never shared.
Key organizations involved in the database creation are NCMEC (National Center for Missing & Exploited Children) and IWF (Internet Watch Foundation), which lead efforts to collect, verify, and catalogue CSAM.
NCMEC: Leading CSAM database in the USA
As America's primary child protection organization, NCMEC creates the foundation for CSAM detection systems used globally. Their database development process includes:
Operating the CyberTipline for public and service provider reporting
Manual verification of reported content by analysts
Converting verified content into digital hashes for secure storage and detection
IWF: Global CSAM prevention leader
The Internet Watch Foundation works internationally with governments, law enforcement, technology companies, and global hotlines. They assess over 7,000 reports weekly, making them one of the world's leading CSAM analysis organizations. More than 190 technology companies use IWF's database and services to protect their users and staff.
They collaborate with technology providers, law enforcement agencies, and governments to create detection systems that platforms can integrate.
Characteristics of known CSAM
Already reported and verified by organizations such as NCMEC and IWF.
Stored as hashes
Relatively easier to detect using hash-matching technology, as these materials are already catalogued.
Represents the majority of CSAM detected and circulated online.
Unknown CSAM
While known CSAM can be identified through hash databases, unknown CSAM presents unique challenges. This category includes newly created or uncatalogued abusive material that hasn't yet been identified, hashed, or documented. Since these materials aren't present in existing databases and often indicate active abuse, their detection requires urgent attention.
Unknown CSAM requires sophisticated artificial intelligence (AI) and machine learning (ML) systems for efficient detection. Key detection components include:
Classifiers: Algorithms trained to sort data into categories by recognizing patterns (e.g., nudity, age, colors, facial features).
AI-powered age analysis: Tools to determine age through pattern recognition
Characteristics of unknown CSAM
Materials that have never been reported or catalogued, making them undetectable by hash-matching technologies
Lacks identifiable digital fingerprints, requiring advanced analysis of imagery, video, and text
Often signals ongoing harm to children, demanding rapid response
Requires analysis of visual details like nudity, facial features, and settings, with age determination being particularly challenging
While automated tools flag potential CSAM, human review is necessary to confirm legal classification.
Core technologies used in CSAM classifiers
To detect unknown CSAM, classifiers rely on a combination of advanced technologies, including:
AI-powered visual analysis
AI-powered visual tools detect nudity, faces, colors, and contextual elements in images or videos to flag potentially abusive content. AI models can recognize these patterns even if they don’t match known examples.
Behavioral pattern analysis
Predictive analysis tools monitor user behavior to identify patterns consistent with grooming or other forms of exploitation, such as repetitive interactions with minors or suspicious messaging activity.
Text-based conversation analysis
Conversations related to grooming, exploitation, or the distribution of CSAM often include specific linguistic patterns. Classifiers analyze text to identify suspicious interactions, especially in messaging platforms.
Metadata analysis
By analyzing metadata from shared files (e.g., timestamps, geolocation, file history), classifiers can flag suspicious activity or uncover hidden connections between offenders.
Key considerations
When selecting a CSAM detection solution, organizations need to balance several critical factors:
Detection capabilities: Understanding the difference between novel content detection versus known content matching
Technical integration: Assessing API compatibility with existing systems
Processing requirements: Evaluating computational resources needed for different approaches
Security protocols: Ensuring proper handling and immediate deletion of sensitive content
Scalability: Planning for growth in content volume and processing needs
Popular CSAM detection and scanning tools
CSAM detection tools are specialized software solutions designed to identify, flag, and prevent the circulation of child sexual abuse material across digital platforms. These tools provide essential protection, helping businesses maintain user safety and meet compliance requirements under laws like the UK's Online Safety Act and the EU's Digital Services Act.
Organizations use these tools to proactively manage risks, protect users, and preserve their reputation. The main categories of detection tools include:
1. Hash-based detection systems
Hash-based detection is one of the most widely used methods for identifying known CSAM. These systems rely on hashing technology, which converts a file (image or video) into a unique digital signature or "hash." and then compares its signatures against databases of known harmful content.
PhotoDNA, developed by Microsoft, is the industry standard in this category. It creates a unique digital signature for images that remains consistent even if the image is slightly modified.
2. AI/ML-based detection tools
Artificial intelligence and machine learning tools focus on detecting unknown CSAM by analyzing patterns, behaviors, and visual elements. These systems use classifiers trained on CSAM datasets to identify potentially abusive content, detecting:
Nudity, explicit imagery, and specific visual patterns
Text-based conversations that suggest grooming or exploitation.
Abuse-related metadata such as suspicious geotags or file information
3. Integrated platform solutions
Some tools combine multiple detection methods (hash-based, AI/ML-based, and metadata analysis) into a single platform, offering end-to-end solutions for combating CSAM. These systems provide additional features such as:
Automated reporting to organizations like NCMEC or law enforcement.
Content moderation workflows that streamline the review process.
Analytics and reporting for compliance and transparency.
PhotoDNA: The industry standard in CSAM hash detection
PhotoDNA, developed by Microsoft and Dartmouth College in 2009, is the industry-leading tool for detecting image-based CSAM. Using advanced perceptual hashing technology, it identifies known harmful content while preserving user privacy. Major technology companies and law enforcement agencies worldwide rely on PhotoDNA in their efforts to combat child exploitation.
PhotoDNA creates unique digital fingerprints of images through three key steps:
Grayscale conversion: Images are converted to grayscale to standardize processing
Grid division: The grayscale image is sectioned into a precise grid
Hash generation: Each grid section receives a numerical value based on its characteristics, creating a unique PhotoDNA signature
These signatures remain stable even when images are modified, resized, or cropped. The automated hashing process is irreversible, ensuring original images cannot be reconstructed from their signatures. The system compares these hashes against databases of known illegal content, particularly those maintained by the National Center for Missing and Exploited Children (NCMEC).
Organizations can access PhotoDNA Cloud Service by applying through Microsoft's PhotoDNA application page. To ensure responsible usage, Microsoft issues API keys only to vetted organizations that meet their approval criteria.
Key features
Resilient detection: Identifies modified versions of known CSAM
Free access: Available at no cost to qualified organizations
Cloud infrastructure: Uses Microsoft Azure for secure, high-speed processing
API integration: Provides REST API access for approved organization
Best for
PhotoDNA is ideal for platforms that need
Accurate, trusted image detection
Large-scale CSAM prevention
Privacy-preserving security measures.
IWF: Global leadership in CSAM prevention and detection
The Internet Watch Foundation (IWF) is a leading global nonprofit organization committed to CSAM from the internet. Through partnerships with governments, law enforcement agencies, and technology companies worldwide, IWF provides comprehensive solutions for detecting and preventing the spread of CSAM.
IWF's effectiveness stems from its multi-faceted strategy:
International collaboration: Partnerships with governments, law enforcement, and over 190 technology companies worldwide
Expert analysis: Professional analysts review more than 7,000 reports weekly, identifying and removing illegal content at a rate of one instance every two minutes
Preventive measures: Development of specialized tools to prevent content re-upload and block access across networks
IWF provides a range of specialized services designed to help organizations detect, block, and prevent the spread of CSAM. These services include:
Tool | Description |
---|---|
Image hash list
| Comprehensive database of images containing CSAM digital fingerprints. Enables platforms to identify and block known harmful content.
|
Keywords list
| Curated collection of terms, phrases, and codes used to conceal CSAM. Supports detection of disguised abusive content
|
URL list
| Dynamic database of verified CSAM-hosting webpages. Allows platforms to block access and prevent content distribution.
|
Non-photographic content list
| Tracks computer-generated, drawn, and animated abuse content. Helps platforms block artificial depictions of abuse.
|
Google's child safety toolkit for CSAM detection and prevention
Google and YouTube provide two specialized tools for detecting CSAM: the Content Safety API and CSAI Match. Offered free to qualified partners, these tools help organizations identify and prevent the spread of abusive content while protecting user privacy and moderator wellbeing.
Content safety API
The Content safety API uses artificial intelligence to detect previously unknown CSAM. Unlike hash-matching tools that identify known content, this API helps platforms discover and address new instances of abuse. It's particularly valuable for:
Organizations processing large volumes of user-generated content
Platforms needing to identify previously uncatalogued CSAM
Services requiring AI-powered content prioritization
Teams seeking to reduce moderator exposure to harmful content
CSAI match
CSAI Match is YouTube's specialized technology for detecting known CSAM videos through hash-matching. This tool is specifically designed for:
Video-sharing platforms and services
Organizations handling high volumes of video content
Platforms requiring scalable video fingerprinting
The system leverages YouTube's extensive database of known CSAM fingerprints, making it a powerful complement to image-focused tools like PhotoDNA.
Shield by project arachnid
Shield by project arachnid is a powerful, API-driven solution developed by Project Arachnid to help electronic service providers (ESPs) proactively detect and remove child sexual abuse material (CSAM) and other harmful-abusive images of children. By integrating this tool into their content moderation strategies, platforms can efficiently prevent the posting and distribution of abusive material.
Shield uses image and video hashing technology, enabling platforms to compare media files against a comprehensive database of known CSAM. This includes both exact matches (unaltered images or videos) and close matches (images or videos with minor modifications), using perceptual hashing to detect altered or derivative content.
The system's effectiveness is demonstrated through its significant operational metrics:
Processed over 171 billion images
Identified more than 93 million suspect media files for expert review
Initiated over 40 million takedown notices to hosting providers
Key features
Free access: Available at no cost to qualifying organizations
Proactive detection: Enables prevention rather than reactive removal
Comprehensive coverage: Detects both unmodified and altered CSAM
Integration flexibility: Works within existing content moderation systems
Safer by Thorn: Comprehensive platform protection against CSAM
Safer by Thorn offers an advanced suite of child protection tools that help platforms detect, review, and report child sexual abuse material (CSAM). Developed by child safety technology experts and trusted by major platforms like VSCO, Vimeo, Bluesky, and Slack, Safer combines innovative detection methods with extensive databases to create a comprehensive safety solution.
Safer's effectiveness is demonstrated through its operational metrics:
130B+ files processed globally.
57M+ known CSAM hashes in the database.
5M+ potential CSAM files flagged on customer platforms since 2019.
1.9M+ files classified as potential CSAM for further action.
Core features of Safer
Safer match: Hash-based detection
Utilizes both cryptographic and perceptual hashing to identify known CSAM
Provides access to over 57.3 million verified CSAM hashes from trusted sources
Features proprietary scene-sensitive video hashing that analyzes individual frames and scenes for precise detection
Safer predict: AI-powered prevention
Employs machine learning to identify previously unreported CSAM
Includes text analysis tools that detect potential grooming conversations
Generates risk scores to help prioritize content review
Enables proactive intervention before harmful content spreads
Moderator-focused review system
Safer's content moderation interface prioritizes both effectiveness and moderator wellbeing through:
Strategic queue management for high-risk content prioritization
Built-in wellness features that minimize unnecessary exposure to harmful content
Streamlined review processes that maintain thorough evaluation standards
Reporting to central authorities
Safer’s reporting tools simplify compliance with local and international reporting obligation
Pre-configured reporting templates for U.S. and Canadian authorities
Secure evidence preservation systems
Centralized reporting channels for swift response.
Cross-platform contribution
Safer enables platforms to collaborate in combating CSAM by facilitating cross-platform sharing of hash values.
Allows platforms to share self-managed hash lists, accelerating detection across ecosystems.
Immediate sharing of new CSAM hash values prevents delays in detection and enforcement.
Hive moderation
Hive has recently expanded its moderation capabilities by integrating a state-of-the-art CSAM detection filter in collaboration with Thorn. This filter leverages embeddings to detect novel child sexual abuse material (CSAM) content in both images and videos. Embeddings are computer-generated scores between 0 and 1 that represent the content’s key characteristics.
How does it work?
When a user uploads an image or video, Hive processes the content through its CSAM detection system. The system generates embeddings, unique numerical representations of the media. These embeddings capture the essential features of the content without retaining any identifiable information. The classifier uses the generated embeddings to evaluate whether the media is likely to be CSAM.
The CSAM classifier provides a response object that includes a numerical score, which can be used by platform administrators to take further action. The score is a probability indicating how likely it is that the content is CSAM, based on the classifier's analysis of the embeddings. The response also includes additional metadata, such as the confidence level of the classification, and can be accessed through Hive's API.
ActiveFence
ActiveFence is a leading provider of Trust and Safety solutions, offering cutting-edge technology to detect harmful content, including CSAM. In partnership with industry leaders, ActiveFence has developed AI models capable of identifying newly generated or manipulated CSAM, going beyond traditional database matching.
ActiveFence employs a multimodal detection model with
Text detection: Identifies CSAM-related discussions, sex solicitation, and age estimation in text, using multilingual analysis, keywords, emojis, and GenAI prompt manipulation techniques.
Image and video detection: Uses computer vision algorithms to spot CSAM indicators, including specific body parts and age estimation, in images and videos.
CometChat: Specialized CSAM detection for messaging apps
CometChat offers a specialized solution for detecting and preventing the distribution of Child Sexual Abuse Material (CSAM) in messaging apps. Built with a rule-based moderation engine, CometChat provides pre-built filters to automatically detect CSAM, perform sentiment analysis, and analyze user behavior. These filters help identify harmful conversations, such as grooming, sexual exploitation, and other forms of online abuse.
The platform allows administrators to set automated actions for messages that are flagged as containing CSAM, ensuring rapid response to harmful content. Additionally, it can take actions on user accounts involved in sharing CSAM, such as suspension or reporting to authorities, to ensure the safety of the app’s user base.
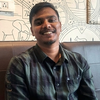
Haris Kumar
Lead Content Strategist , CometChat